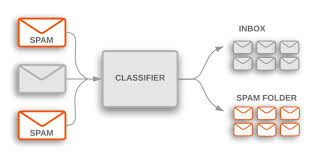
Unlocking Insights: Machine Learning Text Analysis in Action
The Power of Machine Learning in Text Analysis
Text analysis, the process of extracting meaningful insights from written content, has been revolutionized by machine learning. This powerful technology enables computers to learn from data and make predictions or decisions without being explicitly programmed.
Machine learning algorithms can be trained to analyse and interpret vast amounts of text data with incredible speed and accuracy. From sentiment analysis to topic modelling, machine learning has opened up a world of possibilities for understanding and extracting valuable information from text.
Applications of Machine Learning in Text Analysis
Machine learning text analysis is widely used across various industries and fields. In marketing, sentiment analysis helps businesses understand customer opinions and feedback. In healthcare, machine learning algorithms can analyse medical records to assist in diagnosis and treatment planning.
In finance, text analysis can be used to predict market trends by analysing news articles and social media posts. In academia, researchers use machine learning for textual data mining to uncover patterns and insights in research papers and publications.
The Future of Text Analysis with Machine Learning
The field of machine learning text analysis is constantly evolving, with new techniques and algorithms being developed to improve accuracy and efficiency. As more data becomes available, machine learning models will continue to enhance their capabilities in understanding and processing text data.
With the rise of natural language processing (NLP) technologies, machines are becoming increasingly adept at understanding human language nuances, leading to more sophisticated text analysis applications. The future holds exciting possibilities for machine learning in advancing text analysis techniques.
Conclusion
Machine learning has transformed the way we approach text analysis, opening up new opportunities for extracting insights from written content. As technology continues to advance, we can expect even greater advancements in the field of machine learning text analysis, shaping the future of how we understand and interact with textual data.
Understanding Machine Learning Text Analysis: Key Questions and Insights
- What is machine learning text analysis?
- How does machine learning help in analysing text?
- What are the common applications of machine learning in text analysis?
- What are the key challenges in machine learning text analysis?
- How accurate is machine learning in analysing textual data?
- What are the latest advancements in machine learning text analysis?
What is machine learning text analysis?
Machine learning text analysis is a sophisticated approach that utilises machine learning algorithms to extract valuable insights and information from written content. By training computers to understand and interpret text data, machine learning text analysis enables the automation of tasks such as sentiment analysis, topic modelling, and information extraction. This powerful technology allows for the efficient processing of vast amounts of textual data, leading to improved accuracy and speed in analysing and deriving meaning from text. In essence, machine learning text analysis revolutionises the way we approach textual data by leveraging advanced algorithms to uncover patterns, trends, and valuable knowledge embedded within written content.
How does machine learning help in analysing text?
Machine learning plays a crucial role in analysing text by enabling computers to learn patterns and relationships within textual data without explicit programming. Through the use of algorithms and models, machine learning can process large volumes of text efficiently and accurately, extracting valuable insights such as sentiment analysis, topic modelling, and entity recognition. By training on labelled data sets, machine learning algorithms can identify patterns in language usage, categorise text into relevant topics, and even predict future trends based on textual data analysis. Overall, machine learning empowers text analysis by automating the process of extracting meaningful information from text data, leading to more efficient and insightful results.
What are the common applications of machine learning in text analysis?
The common applications of machine learning in text analysis are diverse and impactful across various industries. Machine learning algorithms play a crucial role in sentiment analysis, enabling businesses to understand customer opinions and feedback. In the healthcare sector, machine learning is used to analyse medical records for diagnosis and treatment planning. Financial institutions leverage text analysis to predict market trends by analysing news articles and social media posts. Additionally, researchers in academia utilise machine learning for textual data mining to uncover patterns and insights in research papers and publications. The versatility of machine learning in text analysis continues to drive innovation and enhance decision-making processes across different domains.
What are the key challenges in machine learning text analysis?
In the realm of machine learning text analysis, several key challenges often arise that researchers and practitioners must address. One significant challenge is the issue of data quality and quantity, as obtaining large, high-quality text datasets for training machine learning models can be a daunting task. Another challenge lies in handling the complexity and variability of human language, including nuances, context, and ambiguity, which can pose difficulties for accurate text analysis. Additionally, the interpretability of machine learning models in text analysis is a crucial challenge, as understanding how these models make decisions is essential for ensuring transparency and trustworthiness in their applications. Overcoming these challenges requires continuous innovation and refinement of algorithms to enhance the effectiveness and reliability of machine learning text analysis systems.
How accurate is machine learning in analysing textual data?
The accuracy of machine learning in analysing textual data is a common question that arises when discussing this technology. Machine learning algorithms have shown remarkable capabilities in processing and interpreting vast amounts of text data with high levels of accuracy. However, the accuracy of machine learning models in text analysis can vary depending on various factors such as the quality of the training data, the complexity of the language used, and the specific task being performed. Continuous advancements in machine learning techniques, particularly in natural language processing, are improving the accuracy levels of these models, making them increasingly reliable for extracting valuable insights from textual data.
What are the latest advancements in machine learning text analysis?
One of the frequently asked questions in the field of machine learning text analysis is about the latest advancements in this rapidly evolving domain. Researchers and practitioners are constantly pushing the boundaries of machine learning to enhance text analysis capabilities. Recent advancements include the development of more sophisticated natural language processing models, such as transformer-based architectures like BERT and GPT-3, which have significantly improved language understanding and generation tasks. Additionally, techniques like transfer learning and self-supervised learning have revolutionised how machine learning models are trained on text data, leading to better performance on various text analysis tasks. Overall, the latest advancements in machine learning text analysis are focused on increasing accuracy, efficiency, and interpretability of models to extract deeper insights from textual data.