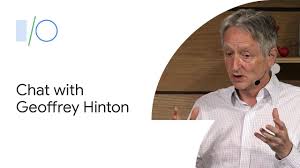
Unveiling the Legacy of Geoffrey Hinton in Deep Learning
Geoffrey Hinton and the Evolution of Deep Learning
A journey through the groundbreaking contributions of a pioneer in artificial intelligence.
Introduction to Geoffrey Hinton
Geoffrey Hinton is widely regarded as one of the founding figures in the field of artificial intelligence, particularly known for his pivotal contributions to deep learning. Born in Wimbledon, London, in 1947, Hinton’s academic journey led him to study at King’s College, Cambridge, and later at the University of Edinburgh, where he earned his PhD in artificial intelligence.
The Birth of Deep Learning
Deep learning is a subset of machine learning that mimics the workings of the human brain in processing data and creating patterns for use in decision-making. Hinton’s work on neural networks laid the foundation for this revolutionary approach. In 1986, along with David Rumelhart and Ronald J. Williams, he published a seminal paper on backpropagation—a method that allows neural networks to learn from their errors.
Breakthroughs and Achievements
Hinton’s research has led to numerous breakthroughs in AI technology. One of his most notable achievements was his involvement with AlexNet, a convolutional neural network developed by his student Alex Krizhevsky. In 2012, AlexNet won the ImageNet Large Scale Visual Recognition Challenge by a significant margin, bringing deep learning into mainstream recognition.
In addition to this achievement, Geoffrey Hinton has been instrumental in developing techniques such as dropout—a method used to prevent overfitting in neural networks—and capsule networks aimed at improving image recognition capabilities.
For his contributions to AI and deep learning, Hinton has received numerous accolades including being named a Fellow of the Royal Society and receiving the prestigious Turing Award in 2018 alongside Yoshua Bengio and Yann LeCun for their work on deep learning.
The Impact on Modern Technology
The impact of Geoffrey Hinton’s work extends far beyond academic circles; it has transformed industries ranging from healthcare to automotive technology. Deep learning algorithms are now integral to voice recognition systems like Siri and Alexa, autonomous vehicles’ navigation systems, medical imaging diagnostics tools, and more.
This transformative impact highlights how foundational research can lead to practical applications that redefine technological capabilities across various sectors.
Geoffrey Hinton: Transformative Contributions to Deep Learning and the Future of AI
- Revolutionized the field of artificial intelligence with groundbreaking contributions to deep learning.
- Pioneered the development of neural networks and backpropagation algorithms, essential components of modern AI systems.
- Instrumental in the creation and advancement of convolutional neural networks, enhancing image recognition capabilities.
- Received prestigious accolades such as the Turing Award for his significant impact on deep learning research.
- Contributed to practical applications of AI technology in diverse industries, from healthcare to autonomous vehicles.
- Continues to inspire new generations of researchers and enthusiasts through his innovative work and dedication to AI advancements.
- His research has reshaped how we approach complex problems in machine learning, paving the way for future innovations.
Seven Challenges of Geoffrey Hinton’s Deep Learning: Complexity, Data Dependency, and More
- Complexity
- Data Dependency
- Black Box Nature
- Overfitting
- Training Time
- Hardware Requirements
- Lack of Explainability
Revolutionized the field of artificial intelligence with groundbreaking contributions to deep learning.
Geoffrey Hinton has revolutionised the field of artificial intelligence with his groundbreaking contributions to deep learning, fundamentally altering the way machines process information. By developing techniques such as backpropagation and advancing neural network architectures, Hinton enabled computers to learn from vast datasets in a manner akin to human cognition. His work laid the foundation for significant advancements in AI applications, from image and speech recognition to natural language processing. These innovations have not only enhanced computational efficiency but also expanded the potential for AI technologies across various industries, marking a pivotal shift in how intelligent systems are designed and implemented.
Pioneered the development of neural networks and backpropagation algorithms, essential components of modern AI systems.
Geoffrey Hinton’s pioneering work in the development of neural networks and backpropagation algorithms has been instrumental in shaping modern artificial intelligence systems. By introducing these foundational concepts, Hinton enabled machines to learn from data in a manner akin to human cognition, revolutionising the way AI systems process information. Neural networks, inspired by the structure of the human brain, allow for complex pattern recognition and decision-making processes. Backpropagation, a method for training these networks by adjusting weights through error minimisation, has become a cornerstone technique in machine learning. Together, these innovations have laid the groundwork for advancements across numerous fields, including computer vision, natural language processing, and autonomous systems, making Hinton’s contributions indispensable to contemporary AI technology.
Instrumental in the creation and advancement of convolutional neural networks, enhancing image recognition capabilities.
Geoffrey Hinton has been instrumental in the creation and advancement of convolutional neural networks, significantly enhancing image recognition capabilities. His pioneering work in developing these networks has revolutionised the field of computer vision, enabling machines to effectively analyse and interpret visual data with remarkable accuracy. Through his contributions, Hinton has paved the way for innovative applications in image processing, object detection, and pattern recognition, shaping the future of artificial intelligence and its impact on various industries.
Received prestigious accolades such as the Turing Award for his significant impact on deep learning research.
Geoffrey Hinton’s profound contributions to the field of deep learning have earned him prestigious accolades, most notably the Turing Award, often referred to as the “Nobel Prize of Computing.” This recognition is a testament to his pioneering work and significant impact on artificial intelligence research. Hinton’s innovative ideas and groundbreaking developments in neural networks have not only advanced academic understanding but have also led to practical applications that are transforming industries worldwide. His receipt of such esteemed honours underscores the importance and far-reaching implications of his research in shaping the future of technology.
Contributed to practical applications of AI technology in diverse industries, from healthcare to autonomous vehicles.
Geoffrey Hinton’s contributions to deep learning have significantly advanced the practical applications of AI technology across a wide array of industries. In healthcare, his work has facilitated the development of sophisticated diagnostic tools that utilise neural networks to analyse medical images with remarkable accuracy, aiding in early detection and treatment of diseases. Meanwhile, in the automotive sector, Hinton’s research underpins the AI systems that power autonomous vehicles, enabling them to navigate complex environments safely and efficiently. Beyond these fields, his innovations have also enhanced technologies such as natural language processing and image recognition, which are integral to everyday applications like virtual assistants and security systems. Hinton’s pioneering efforts have not only expanded the capabilities of AI but have also laid the groundwork for its integration into diverse sectors, transforming how industries operate and innovate.
Continues to inspire new generations of researchers and enthusiasts through his innovative work and dedication to AI advancements.
Geoffrey Hinton’s profound impact on the field of deep learning continues to inspire new generations of researchers and enthusiasts worldwide. His innovative work and unwavering dedication to advancing artificial intelligence have set a high standard for excellence in the industry. By pushing the boundaries of what is possible in AI, Hinton serves as a guiding light for those who seek to explore the vast potential of technology and drive forward the evolution of intelligent systems.
His research has reshaped how we approach complex problems in machine learning, paving the way for future innovations.
Geoffrey Hinton’s research has fundamentally transformed the way complex problems are approached in machine learning, establishing a new paradigm that has paved the way for future innovations. By pioneering deep learning techniques, Hinton introduced methods that allow machines to process vast amounts of data and recognise patterns with unprecedented accuracy. This shift has enabled advancements in various fields, from natural language processing to computer vision, where traditional algorithms struggled to perform effectively. His work on neural networks and backpropagation has not only enhanced the capabilities of AI systems but also inspired a wave of research that continues to push the boundaries of what is possible in artificial intelligence today. As a result, Hinton’s contributions have laid a robust foundation for ongoing developments and breakthroughs in technology.
Complexity
One notable drawback of Geoffrey Hinton’s deep learning approach is the inherent complexity of the models involved, demanding substantial computational resources for both training and deployment. The intricate nature of deep learning algorithms often necessitates powerful hardware and extensive processing capabilities, posing a challenge for organisations with limited resources or budget constraints. This complexity can hinder the widespread adoption of deep learning technologies, as it requires a substantial investment in infrastructure and expertise to effectively harness the potential of these sophisticated models.
Data Dependency
One significant drawback of Geoffrey Hinton’s deep learning approach is its heavy reliance on data dependency. Deep learning algorithms typically demand extensive sets of labelled data to achieve optimal training results, a requirement that can pose challenges as such datasets may not always be easily accessible or readily available. This limitation in data availability can hinder the efficiency and effectiveness of deep learning models, potentially restricting their practical application in scenarios where acquiring large labelled datasets is impractical or costly. Addressing this con remains a critical area of focus for researchers seeking to enhance the scalability and adaptability of deep learning technology.
Black Box Nature
One of the significant criticisms of Geoffrey Hinton’s deep learning models is their “black box” nature, which refers to the difficulty in interpreting and understanding how these models arrive at specific decisions. While deep learning algorithms excel in processing vast amounts of data and identifying complex patterns, they often lack transparency in their decision-making processes. This opacity poses challenges, particularly in critical applications such as healthcare and finance, where understanding the rationale behind a decision is crucial for trust and accountability. The inability to fully decipher the inner workings of these models can lead to issues in debugging, compliance with regulations, and addressing biases, making it imperative for researchers to develop methods that enhance interpretability without compromising performance.
Overfitting
One of the significant challenges associated with Geoffrey Hinton’s deep learning models is the issue of overfitting. Overfitting occurs when a model learns the training data too well, capturing noise and specific patterns that do not generalise to new, unseen data. This means that while the model may exhibit excellent performance on the data it was trained on, its ability to make accurate predictions or classifications on different datasets diminishes. The complexity and flexibility of deep learning models, which allow them to learn intricate patterns in data, also make them susceptible to this problem. Addressing overfitting requires careful consideration of techniques such as regularisation, dropout methods, and cross-validation to ensure that models maintain their predictive power across diverse datasets.
Training Time
One of the notable challenges associated with Geoffrey Hinton’s deep learning models is the significant training time required, particularly when dealing with large-scale datasets. The complexity and depth of these models necessitate substantial computational resources and extended periods for training, which can result in delays when deploying them in real-world applications. This prolonged training time can be a bottleneck for industries that require rapid model iteration and deployment to keep pace with dynamic environments or market demands. Consequently, organisations must invest in high-performance computing infrastructure or explore optimisation techniques to mitigate these delays, which can increase costs and resource allocation efforts.
Hardware Requirements
One notable drawback of Geoffrey Hinton’s deep learning approach is the significant hardware requirements it entails. Implementing and running deep learning algorithms efficiently often demands high-performance hardware infrastructure, which can result in increased costs for organisations and individuals. The need for advanced GPUs, large amounts of memory, and high-speed processors to handle complex computations poses a barrier for those with limited resources, potentially limiting widespread adoption and accessibility of deep learning technology.
Lack of Explainability
A significant drawback of Geoffrey Hinton’s deep learning models is the lack of explainability they offer. The intricate architecture of these models often makes it challenging to comprehend the reasoning behind their predictions or decisions. This lack of transparency can be a critical issue, especially in sensitive areas such as healthcare or finance, where understanding the rationale behind AI-driven outcomes is crucial for trust and accountability. Addressing this con remains a pressing challenge in the field of deep learning, as researchers strive to develop more interpretable models without compromising their performance.