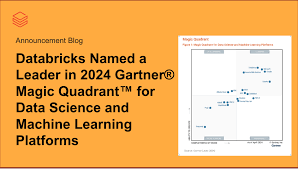
Exploring the Gartner Magic Quadrant for Data Science and Machine Learning in 2022
Gartner Magic Quadrant Data Science and Machine Learning 2022
The Gartner Magic Quadrant is a renowned research methodology that provides a graphical representation of a market’s direction, maturity, and participants. In the realm of data science and machine learning, the Gartner Magic Quadrant serves as a valuable resource for businesses looking to evaluate technology providers in these domains.
As we step into 2022, the Gartner Magic Quadrant for Data Science and Machine Learning is set to offer insights into the evolving landscape of these technologies. With the rapid advancements in AI, automation, and predictive analytics, organisations are increasingly turning to data science and machine learning solutions to drive innovation and gain a competitive edge.
Gartner’s evaluation criteria for the Magic Quadrant typically include completeness of vision and ability to execute. Vendors are positioned within the quadrant based on their performance against these criteria, with leaders demonstrating both strong vision and execution capabilities.
Businesses can leverage the Gartner Magic Quadrant report to identify key players in the data science and machine learning space, understand their strengths and weaknesses, and make informed decisions when selecting technology partners. The report offers valuable insights into market trends, emerging technologies, and best practices that can help organisations stay ahead in today’s data-driven world.
As we navigate through 2022, keeping an eye on the Gartner Magic Quadrant for Data Science and Machine Learning will be essential for businesses seeking to harness the power of data analytics and AI. By staying informed about industry trends and leading vendors in this space, organisations can position themselves for success in an increasingly competitive market.
Exploring Key Insights and Trends: Gartner Magic Quadrant, Data Science, and Machine Learning 2022
- What is Magic Quadrant Gartner 2023?
- What happened to the Gartner DLP Magic Quadrant?
- Is the Gartner Magic Quadrant obsolete?
- What are the trends in data science 2023?
- What are the 4 quadrants of Gartner?
- What is the best platform for machine learning?
- What is the AI hype cycle 2022?
What is Magic Quadrant Gartner 2023?
One frequently asked question regarding the Gartner Magic Quadrant for Data Science and Machine Learning in 2022 is, “What is Magic Quadrant Gartner 2023?” As businesses look ahead to the next year, they are curious about how the landscape of data science and machine learning technologies will evolve. The anticipation for the Gartner Magic Quadrant report for 2023 is high, as it is expected to provide valuable insights into emerging trends, innovative solutions, and key players shaping the future of data analytics and AI. Organisations are eager to learn about the advancements in technology and market dynamics that will be highlighted in the upcoming report, as they continue to leverage data-driven strategies for growth and competitive advantage.
What happened to the Gartner DLP Magic Quadrant?
The Gartner Data Loss Prevention (DLP) Magic Quadrant has been integrated into the broader Gartner Magic Quadrant for Data Science and Machine Learning in 2022. This move reflects the evolving landscape of data security and the increasing importance of incorporating DLP capabilities within the broader context of data science and machine learning technologies. By consolidating DLP evaluation within the larger framework of data science and machine learning, Gartner aims to provide a more comprehensive assessment of vendors’ capabilities in addressing data protection challenges in today’s digital age.
Is the Gartner Magic Quadrant obsolete?
The question of whether the Gartner Magic Quadrant is obsolete is a common one in discussions surrounding data science and machine learning in 2022. While some critics argue that the traditional quadrant model may not fully capture the complexities of today’s rapidly evolving technology landscape, many industry experts still find value in the insights provided by Gartner’s analysis. As technology continues to advance at a rapid pace, it is crucial for organisations to adapt their evaluation methods and consider multiple sources of information when making strategic decisions. While the Gartner Magic Quadrant may not be perfect, it remains a valuable tool for assessing vendors and understanding market trends in the dynamic field of data science and machine learning.
What are the trends in data science 2023?
In 2023, the field of data science is experiencing several key trends that are shaping the industry. One prominent trend is the increasing adoption of automated machine learning (AutoML) tools, which streamline and democratise the data science process by allowing users with varying expertise to build effective models. Additionally, there is a growing emphasis on ethical AI and responsible data usage, as organisations strive to ensure transparency and fairness in their algorithms. The integration of advanced analytics with real-time data processing is also becoming more prevalent, enabling businesses to make quicker, more informed decisions. Furthermore, the rise of edge computing is facilitating data processing closer to the source, reducing latency and improving efficiency. These trends highlight a shift towards more accessible, ethical, and efficient data science practices in 2023.
What are the 4 quadrants of Gartner?
The Gartner Magic Quadrant is structured into four quadrants: Leaders, Challengers, Visionaries, and Niche Players. These quadrants represent different categories of technology providers based on their completeness of vision and ability to execute. Leaders are positioned in the top-right quadrant and are recognised for their strong performance in both vision and execution. Challengers may excel in execution but might lack a comprehensive vision. Visionaries demonstrate innovative ideas and future potential but may need to improve their execution capabilities. Niche Players focus on specific market segments or niche offerings, showcasing expertise in a particular area. Understanding these four quadrants helps businesses navigate the Gartner Magic Quadrant report effectively and make informed decisions when evaluating technology solutions for data science and machine learning in 2022.
What is the best platform for machine learning?
In the realm of data science and machine learning, the question of “What is the best platform for machine learning?” is a common inquiry that many businesses and professionals seek to address. The Gartner Magic Quadrant report for Data Science and Machine Learning 2022 serves as a valuable resource for identifying top platforms in this space. By evaluating vendors based on their completeness of vision and ability to execute, the report offers insights into leading platforms that excel in providing robust tools, scalability, performance, and support for machine learning initiatives. Organisations can leverage this information to make informed decisions when selecting a platform that aligns with their specific requirements and strategic goals in the ever-evolving landscape of data science and machine learning.
What is the AI hype cycle 2022?
The AI Hype Cycle 2022, as outlined by Gartner, provides a comprehensive overview of the maturity and adoption of various artificial intelligence technologies. It serves as a visual representation that tracks the progression of AI innovations from initial conception through to mainstream adoption. The cycle typically includes stages such as the “Innovation Trigger,” where new technologies emerge; the “Peak of Inflated Expectations,” where hype often exceeds reality; the “Trough of Disillusionment,” where interest wanes due to unmet expectations; the “Slope of Enlightenment,” where practical benefits start to become apparent; and finally, the “Plateau of Productivity,” where technologies become widely adopted and deliver tangible benefits. For organisations navigating the AI landscape in 2022, understanding this cycle helps in making informed decisions about which technologies to invest in and when, ensuring they align with realistic expectations and strategic goals.