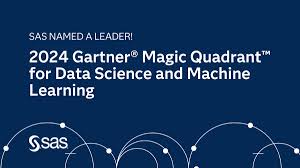
Navigating the Gartner Magic Quadrant for Data Science & Machine Learning Platforms: A Comprehensive Guide
The Gartner Magic Quadrant for Data Science & Machine Learning Platforms: A Comprehensive Overview
The Gartner Magic Quadrant is a renowned research methodology that evaluates technology providers based on their completeness of vision and ability to execute in a specific market. In the realm of data science and machine learning platforms, the Gartner Magic Quadrant serves as a valuable resource for businesses and professionals looking to make informed decisions about technology investments.
Each year, Gartner releases its Magic Quadrant report for Data Science & Machine Learning Platforms, providing an in-depth analysis of the key players in the industry. The report categorises vendors into four quadrants:
- Leaders: Companies that demonstrate a strong vision and execution capabilities, often leading the market in innovation and customer satisfaction.
- Challengers: Providers with solid capabilities but may lack the same level of vision as Leaders.
- Visionaries: Companies that exhibit a clear vision but may face challenges in executing their strategies.
- Niche Players: Specialised vendors that focus on specific niches or emerging technologies.
By examining the Gartner Magic Quadrant for Data Science & Machine Learning Platforms, businesses can gain insights into which vendors are best positioned to meet their specific needs. The report considers factors such as product functionality, customer feedback, market presence, and overall strategy to assess each vendor’s position within the quadrant.
For organisations seeking data science and machine learning solutions, leveraging the insights provided by the Gartner Magic Quadrant can help streamline the vendor selection process and drive successful technology implementations. By aligning business requirements with vendor capabilities identified in the report, companies can make informed decisions that support their long-term goals.
In conclusion, the Gartner Magic Quadrant for Data Science & Machine Learning Platforms offers a comprehensive overview of the competitive landscape within this rapidly evolving industry. By utilising this valuable resource, organisations can navigate the complex technology market with confidence and position themselves for success in an increasingly data-driven world.
Key Considerations for Navigating the Gartner Magic Quadrant in Data Science and Machine Learning Platforms
- Understand the criteria used by Gartner to evaluate data science and machine learning platforms.
- Consider both the ‘Ability to Execute’ and ‘Completeness of Vision’ dimensions in the Magic Quadrant.
- Look beyond just the Leaders quadrant – niche players may also offer solutions that fit your specific needs.
- Evaluate how well a platform aligns with your organisation’s goals and technical requirements.
- Take into account customer reviews, case studies, and analyst insights in addition to the Magic Quadrant placement.
- Regularly monitor updates to the Magic Quadrant as positions can change based on market trends and vendor performance.
Understand the criteria used by Gartner to evaluate data science and machine learning platforms.
To make the most of the Gartner Magic Quadrant for Data Science & Machine Learning Platforms, it is crucial to grasp the criteria employed by Gartner in assessing these technologies. Understanding the specific benchmarks and metrics that Gartner uses to evaluate data science and machine learning platforms can provide valuable insights into the strengths and weaknesses of each vendor. By familiarising oneself with these criteria, businesses and professionals can make well-informed decisions when selecting a platform that aligns with their unique requirements and strategic objectives.
Consider both the ‘Ability to Execute’ and ‘Completeness of Vision’ dimensions in the Magic Quadrant.
When evaluating vendors in the Gartner Magic Quadrant for Data Science & Machine Learning Platforms, it is crucial to consider both the ‘Ability to Execute’ and ‘Completeness of Vision’ dimensions. The ‘Ability to Execute’ dimension assesses a vendor’s capability to deliver on its promises, meet customer needs, and execute its product roadmap effectively. On the other hand, the ‘Completeness of Vision’ dimension evaluates a vendor’s innovation, strategic direction, and understanding of market trends. By examining both dimensions together, businesses can gain a holistic view of a vendor’s strengths and weaknesses, enabling them to make well-informed decisions that align with their specific requirements and long-term goals.
Look beyond just the Leaders quadrant – niche players may also offer solutions that fit your specific needs.
When exploring the Gartner Magic Quadrant for Data Science & Machine Learning Platforms, it is essential to look beyond just the Leaders quadrant. While Leaders often represent established market players with comprehensive offerings, niche players may offer tailored solutions that precisely fit your specific needs. These specialised vendors bring unique expertise and innovative approaches to the table, addressing niche requirements that may not be fully covered by larger providers. By considering niche players alongside Leaders, businesses can uncover hidden gems that offer targeted solutions and potentially unlock significant value for their data science and machine learning initiatives.
Evaluate how well a platform aligns with your organisation’s goals and technical requirements.
When considering the Gartner Magic Quadrant for Data Science & Machine Learning Platforms, it is crucial to evaluate how well a platform aligns with your organisation’s goals and technical requirements. By carefully assessing whether a platform can meet your specific business objectives and technical needs, you can make an informed decision that maximises the value of your technology investment. Understanding the alignment between the platform’s capabilities and your organisation’s strategic priorities is key to selecting a solution that will support your long-term success in data science and machine learning initiatives.
Take into account customer reviews, case studies, and analyst insights in addition to the Magic Quadrant placement.
When considering the Gartner Magic Quadrant for Data Science & Machine Learning Platforms, it is essential to broaden the scope beyond just the quadrant placement. Valuable insights can be gained by delving into customer reviews, exploring real-world case studies, and considering analyst perspectives. By incorporating these additional sources of information, businesses can gain a more comprehensive understanding of how a particular platform performs in practical scenarios and how it aligns with their specific requirements. Customer feedback, success stories, and expert analysis provide a holistic view that complements the Magic Quadrant assessment, enabling informed decision-making and ensuring that the chosen platform meets both current needs and future objectives.
Regularly monitor updates to the Magic Quadrant as positions can change based on market trends and vendor performance.
It is crucial to regularly monitor updates to the Gartner Magic Quadrant for Data Science & Machine Learning Platforms as positions within the quadrant can shift based on evolving market trends and vendor performance. By staying informed about these updates, businesses can adapt their technology strategies to align with the latest industry developments and ensure that they are leveraging the most innovative and effective solutions available. Keeping a close eye on changes in vendor positions can help organisations make informed decisions when selecting data science and machine learning platforms that best suit their evolving needs and objectives.