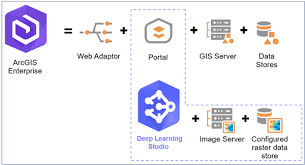
Exploring the Power of Deep Learning in ArcGIS Pro
Unlocking the Potential of Spatial Analysis: ArcGIS Pro and Deep Learning
The integration of deep learning with geographic information systems (GIS) has opened up new vistas for spatial analysis and interpretation. ArcGIS Pro, Esri’s powerful mapping and analytics software, is at the forefront of this integration, providing tools that harness the capabilities of deep learning to transform vast amounts of spatial data into actionable insights.
What is Deep Learning?
Deep learning is a subset of machine learning where artificial neural networks—algorithms inspired by the human brain—learn from large amounts of data. These networks can identify patterns and features in data that are too complex for traditional data analysis techniques.
Deep Learning in ArcGIS Pro
ArcGIS Pro offers a suite of deep learning tools that enable users to perform sophisticated spatial analyses such as object detection, image classification, and feature extraction from geospatial imagery. By leveraging deep learning models within ArcGIS Pro, users can automate processes that were once time-consuming and improve the accuracy of their results.
Object Detection and Image Classification
With deep learning tools in ArcGIS Pro, users can train models to recognize specific objects within satellite images or aerial photography. This capability is crucial for urban planning, environmental monitoring, and disaster response. For instance, models can be trained to identify buildings damaged by natural disasters or to classify land use patterns over large regions.
Feature Extraction
Feature extraction involves identifying and delineating specific features within imagery—such as roads, vegetation types or bodies of water—and converting them into vector layers for further analysis. Deep learning models significantly enhance the efficiency and precision with which these features can be extracted.
The Workflow: From Imagery to Insights
The deep learning workflow in ArcGIS Pro typically involves several steps:
- Data Preparation: Gathering and pre-processing imagery datasets suitable for training the model.
- Model Training: Selecting an appropriate neural network architecture and using a subset of the dataset to train the model on recognising specific patterns or objects.
- Inference: Applying the trained model to new datasets to predict or classify data points automatically.
- Validation: Assessing the model’s accuracy by comparing its predictions against known outcomes or expert analysis.
- Actionable Insights: Integrating the results back into GIS for visualization, further analysis, decision-making processes or policy development.
The Future Is Now: Embracing AI in GIS
The incorporation of AI through deep learning marks a significant shift in how spatial data is analysed. As these technologies continue to evolve, they will offer even more sophisticated tools for professionals across various industries—from urban planning to conservation biology—to make informed decisions based on comprehensive spatial analyses provided by platforms like ArcGIS Pro.
ArcGIS Pro’s commitment to integrating AI ensures that GIS professionals will remain at the cutting edge of spatial science technology. With ongoing updates and support from Esri’s extensive knowledge base, users can expect continual improvements in their capacity to harness deep learning for groundbreaking work in their respective fields.
Maximising Deep Learning Potential in ArcGIS Pro: 8 Essential Tips for Advanced Geospatial Analysis
- Ensure your ArcGIS Pro software is up-to-date to access the latest deep learning tools.
- Familiarise yourself with the deep learning capabilities in ArcGIS Pro through online tutorials and documentation.
- Prepare high-quality training data sets for accurate deep learning model training.
- Experiment with different deep learning algorithms to find the most suitable one for your specific task.
- Regularly validate and fine-tune your deep learning models to improve their performance over time.
- Consider using transfer learning techniques to leverage pre-trained models for faster and more efficient training.
- Collaborate with colleagues or join online communities to share knowledge and best practices in ArcGIS Pro deep learning.
- Stay updated on advancements in deep learning technology to continuously enhance your skills and workflows.
Ensure your ArcGIS Pro software is up-to-date to access the latest deep learning tools.
To fully utilise the deep learning capabilities within ArcGIS Pro, it is essential to ensure that your software is kept up-to-date. By regularly updating your ArcGIS Pro software, you can access the latest advancements and tools in deep learning that Esri provides. Staying current with updates not only enhances the performance and functionality of the software but also enables you to leverage cutting-edge features for more accurate and efficient spatial analyses. Keeping your ArcGIS Pro software up-to-date is key to unlocking the full potential of deep learning in your GIS projects.
Familiarise yourself with the deep learning capabilities in ArcGIS Pro through online tutorials and documentation.
To maximise your proficiency in utilising deep learning within ArcGIS Pro, it is advisable to acquaint yourself with the software’s capabilities by exploring online tutorials and documentation. These valuable resources provide step-by-step guidance on how to leverage deep learning tools effectively, enabling you to enhance your spatial analysis skills and derive actionable insights from geospatial data. By immersing yourself in these resources, you can gain a deeper understanding of ArcGIS Pro’s deep learning functionalities and unlock its full potential for transforming complex spatial datasets into meaningful outcomes.
Prepare high-quality training data sets for accurate deep learning model training.
To maximise the accuracy and effectiveness of deep learning model training in ArcGIS Pro, it is essential to prepare high-quality training data sets. The quality of the data used directly impacts the model’s ability to recognise patterns and features within geospatial imagery. By ensuring that training data sets are comprehensive, relevant, and accurately labelled, users can enhance the performance of their deep learning models, leading to more precise object detection, image classification, and feature extraction results. Investing time and effort in preparing high-quality training data sets is a crucial step towards achieving reliable and actionable insights through deep learning in ArcGIS Pro.
Experiment with different deep learning algorithms to find the most suitable one for your specific task.
To maximise the potential of ArcGIS Pro’s deep learning capabilities, it is recommended to experiment with various deep learning algorithms to identify the most effective one for your particular task. By exploring different algorithms, you can determine which one best suits the complexities and nuances of your spatial analysis requirements. This iterative approach allows you to fine-tune your models and achieve optimal results, ensuring that you leverage the full power of deep learning within ArcGIS Pro for accurate and insightful analyses.
Regularly validate and fine-tune your deep learning models to improve their performance over time.
Regularly validating and fine-tuning your deep learning models in ArcGIS Pro is essential to ensure their continued effectiveness and accuracy in spatial analysis. By periodically assessing the model’s performance against new data and making adjustments based on these evaluations, you can enhance its predictive capabilities and maintain its relevance as spatial patterns evolve over time. This iterative process of validation and refinement not only optimises the model’s performance but also allows for ongoing improvements that align with the dynamic nature of geospatial data, ultimately leading to more robust and reliable insights for your GIS projects.
Consider using transfer learning techniques to leverage pre-trained models for faster and more efficient training.
When delving into deep learning within ArcGIS Pro, it is advisable to explore transfer learning techniques as a means to enhance training efficiency. By utilising pre-trained models and transferring their knowledge to new tasks, users can expedite the training process and achieve more accurate results. This approach not only saves time but also leverages the expertise embedded in existing models, ultimately leading to improved performance in spatial analyses and interpretation within the ArcGIS Pro environment.
Collaborate with colleagues or join online communities to share knowledge and best practices in ArcGIS Pro deep learning.
Collaborating with colleagues or engaging with online communities is a valuable tip for maximising the potential of ArcGIS Pro deep learning. By sharing knowledge and best practices with peers, professionals can gain new insights, troubleshoot challenges more effectively, and stay updated on the latest advancements in the field. Online forums and communities provide a platform for exchanging ideas, learning from others’ experiences, and fostering a supportive network of like-minded individuals passionate about leveraging deep learning in spatial analysis. Embracing collaboration not only enhances individual expertise but also contributes to the collective growth and innovation within the ArcGIS Pro community.
Stay updated on advancements in deep learning technology to continuously enhance your skills and workflows.
To maximise the potential of deep learning within ArcGIS Pro, it is essential to stay abreast of the latest advancements in this rapidly evolving technology. By keeping yourself updated on new developments and techniques in deep learning, you can continuously enhance your skills and workflows. This proactive approach ensures that you remain at the forefront of leveraging cutting-edge tools and methodologies to extract valuable insights from spatial data, ultimately enhancing the efficiency and accuracy of your analyses within ArcGIS Pro.