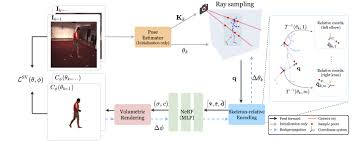
Unleashing the Power of Nerf in Deep Learning: Revolutionizing 3D Scene Reconstruction
The Impact of Nerf on Deep Learning
Neural Radiance Fields (NeRF) have emerged as a groundbreaking technique in the field of deep learning, revolutionizing how we perceive and interact with 3D scenes. NeRF has gained significant attention for its ability to generate high-fidelity, photorealistic 3D reconstructions from 2D images, pushing the boundaries of what is possible with deep learning.
One of the key advantages of Nerf is its ability to capture complex scene geometry and appearance in a single model, eliminating the need for separate rendering and reconstruction steps. This not only streamlines the process but also produces more accurate and detailed results compared to traditional methods.
The integration of Nerf into deep learning frameworks has opened up new possibilities for applications such as virtual reality, augmented reality, and computer graphics. By enabling the generation of immersive 3D environments from simple 2D images, Nerf has the potential to transform how we experience digital content.
Researchers and developers are actively exploring ways to further enhance Nerf’s capabilities and overcome its limitations, such as scalability and computational complexity. As advancements in hardware and algorithms continue to evolve, we can expect Nerf to play an increasingly significant role in shaping the future of deep learning.
In conclusion, Nerf represents a paradigm shift in how we approach 3D scene reconstruction and rendering through deep learning. Its impact on various industries and applications is profound, paving the way for exciting developments in virtual environments and visual content creation.
Exploring Nerf in Deep Learning: Key Questions and Insights
- What is Nerf in the context of deep learning?
- How does Nerf revolutionize 3D scene reconstruction?
- What are the key advantages of using Nerf in deep learning?
- What applications benefit from integrating Nerf into deep learning frameworks?
- What challenges or limitations does Nerf face in practice?
- How can researchers enhance the capabilities of Nerf for better performance?
- In what ways is Nerf transforming virtual reality and augmented reality experiences?
- What role does hardware and algorithms play in advancing the effectiveness of Nerf?
What is Nerf in the context of deep learning?
In the realm of deep learning, Nerf, short for Neural Radiance Fields, is a cutting-edge technique that has garnered significant attention for its ability to revolutionize 3D scene reconstruction and rendering. Nerf represents a powerful neural network architecture that can infer detailed 3D scenes from 2D images with remarkable accuracy and realism. By combining the principles of neural networks and radiance fields, Nerf enables the generation of photorealistic 3D models directly from images, eliminating the need for separate rendering processes. This innovative approach has opened up new avenues for immersive experiences in virtual reality, augmented reality, and computer graphics, making Nerf a game-changer in the field of deep learning.
How does Nerf revolutionize 3D scene reconstruction?
Neural Radiance Fields (Nerf) revolutionize 3D scene reconstruction by offering a groundbreaking approach that combines neural networks with traditional rendering techniques. Unlike conventional methods that rely on separate steps for scene geometry and appearance, Nerf encapsulates both aspects within a single model, enabling the generation of highly detailed and photorealistic 3D reconstructions from 2D images. This integration eliminates the need for complex pre-processing and post-processing steps, streamlining the reconstruction process and producing more accurate results. By leveraging deep learning capabilities, Nerf has fundamentally transformed how we perceive and interact with 3D scenes, pushing the boundaries of what is achievable in the realm of computer vision and graphics.
What are the key advantages of using Nerf in deep learning?
When exploring the key advantages of utilising Neural Radiance Fields (NeRF) in deep learning, it becomes evident that Nerf offers a revolutionary approach to 3D scene reconstruction. One of its primary strengths lies in its capability to generate highly detailed and photorealistic 3D models directly from 2D images, eliminating the need for separate rendering processes. This streamlined approach not only enhances efficiency but also results in more accurate and intricate representations of complex scene geometry and appearance. By integrating Nerf into deep learning frameworks, researchers and developers can unlock new possibilities in virtual reality, augmented reality, and computer graphics, showcasing Nerf’s potential to redefine how we interact with and experience digital content in immersive 3D environments.
What applications benefit from integrating Nerf into deep learning frameworks?
The integration of Neural Radiance Fields (Nerf) into deep learning frameworks offers a wide range of applications that stand to benefit significantly from this innovative technology. Industries such as virtual reality, augmented reality, computer graphics, and digital content creation are among the key beneficiaries of incorporating Nerf into deep learning models. By enabling the generation of highly detailed and immersive 3D environments from 2D images, Nerf enhances the realism and interactivity of virtual experiences. Moreover, Nerf’s ability to capture complex scene geometry and appearance in a single model simplifies the process of rendering and reconstruction, making it a valuable tool for enhancing visual content across various platforms and industries.
What challenges or limitations does Nerf face in practice?
In practice, Neural Radiance Fields (NeRF) face several challenges and limitations that researchers and developers are actively working to address. One significant challenge is the computational complexity of training and inference with NeRF models, which can be resource-intensive and time-consuming, especially for high-resolution scenes. Another limitation is the scalability of NeRF to handle large-scale environments efficiently, as the memory and processing requirements can become prohibitive. Additionally, NeRF may struggle with capturing dynamic or moving scenes effectively due to its reliance on static 3D representations. Despite these challenges, ongoing advancements in hardware capabilities and algorithmic improvements hold promise for overcoming these limitations and unlocking the full potential of NeRF in practical applications.
How can researchers enhance the capabilities of Nerf for better performance?
Researchers are continuously exploring ways to enhance the capabilities of Neural Radiance Fields (Nerf) in order to improve its performance. One approach is to focus on optimizing the computational efficiency of Nerf models to reduce training time and resource requirements. Additionally, refining the neural network architecture used in Nerf models and incorporating advanced techniques such as regularization and data augmentation can help improve the model’s accuracy and generalization capabilities. Furthermore, exploring novel training strategies and loss functions tailored to the specific challenges of Nerf can also contribute to enhancing its performance for generating more realistic and detailed 3D reconstructions from 2D images.
In what ways is Nerf transforming virtual reality and augmented reality experiences?
Nerf (Neural Radiance Fields) is revolutionizing virtual reality and augmented reality experiences in several transformative ways. By leveraging its advanced deep learning capabilities, Nerf enables the creation of highly detailed and photorealistic 3D scenes from 2D images, enhancing the immersion and realism of virtual environments. This breakthrough technology eliminates the need for separate rendering and reconstruction steps, streamlining the process of generating interactive and dynamic AR/VR content. Nerf’s ability to capture complex scene geometry and appearance in a single model allows for more realistic interactions and visualisations, pushing the boundaries of what is achievable in virtual and augmented reality experiences.
What role does hardware and algorithms play in advancing the effectiveness of Nerf?
The role of hardware and algorithms is paramount in advancing the effectiveness of Nerf in deep learning. Hardware capabilities, such as powerful GPUs and specialised processors, are essential for handling the computational demands of training complex neural networks like Nerf. Efficient algorithms play a crucial role in optimising model performance, reducing training time, and enhancing the accuracy of 3D reconstructions. The synergy between cutting-edge hardware and innovative algorithms is key to pushing the boundaries of what Nerf can achieve in terms of generating realistic 3D scenes from 2D images.