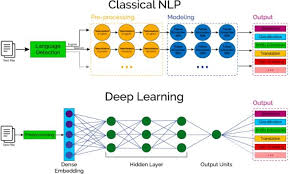
Unveiling the Wonders of Neural Networks and Deep Learning
The Fascinating World of Neural Networks and Deep Learning
Neural networks and deep learning have revolutionised the field of artificial intelligence, paving the way for incredible advancements in various industries. These sophisticated systems are inspired by the way the human brain works, with interconnected nodes that process information and learn from data.
Neural networks consist of layers of neurons that are interconnected through weighted connections. Through a process known as training, these networks can learn to recognise patterns, make predictions, and perform complex tasks with remarkable accuracy.
The Power of Deep Learning
Deep learning takes neural networks to the next level by adding more layers to the network, allowing for more complex computations and representations of data. This depth enables deep learning models to learn intricate features from raw data, leading to breakthroughs in image recognition, natural language processing, autonomous driving, and more.
Applications Across Industries
The applications of neural networks and deep learning are vast and diverse. In healthcare, these technologies are used for medical imaging analysis, disease diagnosis, and drug discovery. In finance, they power algorithmic trading systems and fraud detection mechanisms.
In marketing, neural networks help analyse consumer behaviour and tailor personalised recommendations. In transportation, deep learning algorithms enhance route optimisation and traffic prediction. The possibilities are endless as these technologies continue to evolve.
The Future of AI
As neural networks and deep learning algorithms become more sophisticated and efficient, we can expect further advancements in AI-driven solutions. From self-driving cars to intelligent virtual assistants, the future is bright with possibilities as we harness the power of neural networks and deep learning.
Understanding Neural Networks and Deep Learning: Frequently Asked Questions
- What are neural networks and deep learning?
- How do neural networks work?
- What is the difference between neural networks and deep learning?
- What are the applications of neural networks and deep learning?
- How is deep learning used in image recognition?
- What industries benefit from neural networks and deep learning?
- What are the challenges of implementing deep learning systems?
- How can I learn more about neural networks and deep learning?
What are neural networks and deep learning?
Neural networks are computational models inspired by the human brain’s structure and function, consisting of interconnected nodes or neurons that process information in layers. These networks are designed to recognise patterns and make decisions based on data inputs. Deep learning, a subset of machine learning, builds upon neural networks by adding multiple hidden layers, allowing the model to learn complex representations and features from large datasets. This advanced approach enables deep learning algorithms to achieve remarkable accuracy in tasks such as image and speech recognition, natural language processing, and autonomous systems, significantly advancing the field of artificial intelligence.
How do neural networks work?
Neural networks are complex systems inspired by the human brain’s structure and function. At their core, neural networks consist of interconnected nodes, or artificial neurons, organised in layers. Each neuron processes input data through weighted connections and applies an activation function to produce an output. During training, these connections are adjusted iteratively to minimise errors and improve the network’s ability to make accurate predictions or classifications. In essence, neural networks learn from data by adjusting their internal parameters through a process known as backpropagation, allowing them to recognise patterns, extract features, and perform tasks with increasing proficiency over time.
What is the difference between neural networks and deep learning?
When exploring the realm of neural networks and deep learning, a common question that arises is: What is the difference between neural networks and deep learning? Neural networks refer to interconnected layers of nodes that process information and learn from data through weighted connections. On the other hand, deep learning takes neural networks a step further by adding more layers to enable complex computations and representations of data. In essence, while neural networks form the foundation, deep learning enhances this structure with increased depth for learning intricate features from raw data, leading to significant advancements in various fields.
What are the applications of neural networks and deep learning?
Neural networks and deep learning have a wide range of applications across various industries, showcasing their versatility and power in the field of artificial intelligence. These advanced technologies are utilised in healthcare for tasks such as medical image analysis, disease diagnosis, and drug discovery. In finance, neural networks play a crucial role in algorithmic trading systems and fraud detection mechanisms. Marketing professionals leverage these tools to analyse consumer behaviour and provide personalised recommendations. Additionally, neural networks and deep learning algorithms are instrumental in transportation for route optimisation and traffic prediction. The diverse applications of neural networks and deep learning continue to drive innovation and transform industries worldwide.
How is deep learning used in image recognition?
Deep learning plays a pivotal role in image recognition by leveraging neural networks with multiple layers to extract intricate features from visual data. In the context of image recognition, deep learning models are trained on vast datasets to learn patterns and structures within images. Through this process, the neural network can identify objects, shapes, textures, and other visual elements with remarkable accuracy. By utilising convolutional neural networks (CNNs) and techniques like pooling and activation functions, deep learning algorithms excel at tasks such as object detection, facial recognition, and image classification. The ability of deep learning to automatically learn hierarchical representations of images has revolutionised the field of computer vision and paved the way for advancements in areas like autonomous vehicles, medical imaging analysis, and augmented reality applications.
What industries benefit from neural networks and deep learning?
Neural networks and deep learning have significantly impacted various industries, offering transformative solutions and driving innovation. Industries such as healthcare benefit from these technologies through improved medical imaging analysis, disease diagnosis, and drug discovery. In finance, neural networks power algorithmic trading systems and enhance fraud detection mechanisms. Marketing industries utilise these advanced systems to analyse consumer behaviour and deliver personalised recommendations. Additionally, transportation sectors benefit from deep learning algorithms for route optimisation and traffic prediction. The versatility of neural networks and deep learning continues to revolutionise these industries by enabling more efficient processes and unlocking new possibilities for advancement.
What are the challenges of implementing deep learning systems?
Implementing deep learning systems poses several challenges that developers and researchers often encounter. One major challenge is the need for large amounts of high-quality data to train deep neural networks effectively. Data preprocessing, cleaning, and augmentation are crucial steps in preparing datasets for training, requiring significant time and resources. Additionally, selecting the right architecture, optimising hyperparameters, and managing computational resources efficiently are key challenges in building robust deep learning systems. Furthermore, interpreting the inner workings of complex deep learning models and ensuring their interpretability and transparency remain ongoing challenges in deploying these systems effectively in real-world applications.
How can I learn more about neural networks and deep learning?
For those eager to delve deeper into the realms of neural networks and deep learning, there are various resources available to expand your knowledge and skills in this fascinating field. Consider enrolling in online courses offered by reputable platforms such as Coursera, Udemy, or edX, where you can access structured lessons and hands-on projects guided by experts. Additionally, exploring textbooks like “Deep Learning” by Ian Goodfellow, Yoshua Bengio, and Aaron Courville can provide a comprehensive understanding of the theoretical foundations behind these technologies. Engaging with online communities, attending workshops, and experimenting with open-source frameworks like TensorFlow and PyTorch are also valuable ways to enhance your expertise in neural networks and deep learning. Remember, continuous learning and practical application are key to mastering these innovative concepts.