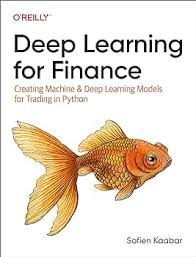
Exploring the Impact of Deep Learning in Finance
The Role of Deep Learning in Revolutionizing Finance
In recent years, deep learning has emerged as a powerful tool in the financial industry, transforming the way businesses operate and make decisions. Deep learning, a subset of artificial intelligence, uses neural networks to mimic the way the human brain processes information. This technology has proven to be highly effective in analysing vast amounts of data and extracting valuable insights that can drive strategic financial decisions.
Applications of Deep Learning in Finance
One of the key applications of deep learning in finance is predictive analytics. By analysing historical data and identifying patterns, deep learning algorithms can forecast market trends, stock prices, and risk factors with remarkable accuracy. Financial institutions use these insights to make informed investment decisions, manage risks effectively, and optimize their portfolios.
Another important application is fraud detection. Deep learning models can detect anomalies in financial transactions and identify potential fraudulent activities in real-time. This helps banks and other financial institutions prevent fraud before it occurs, saving them significant losses and maintaining trust with their customers.
The Future of Deep Learning in Finance
The future of deep learning in finance looks promising as more companies embrace this technology to gain a competitive edge. With advancements in computing power and data availability, deep learning models are becoming more sophisticated and capable of handling complex financial tasks.
Moreover, regulators are also recognizing the potential benefits of deep learning in improving compliance processes and enhancing transparency in the financial industry. As regulations evolve to accommodate new technologies, we can expect to see deeper integration of deep learning tools across various sectors within finance.
Conclusion
Deep learning is revolutionizing the finance industry by providing advanced analytical capabilities that were previously unimaginable. As businesses continue to leverage this technology for better decision-making, we can expect to see increased efficiency, reduced risks, and improved customer experiences across the financial landscape.
Frequently Asked Questions: Deep Learning Applications in Finance and Banking
- What is DL in finance?
- How is deep learning used in banks?
- What is an example of deep learning in banking?
- Is deep learning good for finance?
- What machine learning is used in finance?
- How is deep learning used in finance?
What is DL in finance?
Deep learning in finance refers to the application of advanced artificial intelligence techniques, specifically deep neural networks, to analyse and interpret complex financial data. DL enables financial institutions to extract valuable insights from vast amounts of data, predict market trends, manage risks, detect fraud, and make informed investment decisions. By leveraging deep learning algorithms, businesses in the financial sector can enhance their decision-making processes, improve efficiency, and stay competitive in an ever-evolving market landscape.
How is deep learning used in banks?
Deep learning is utilised in banks to enhance a variety of operations and services, significantly improving efficiency and accuracy. One primary application is in fraud detection, where deep learning algorithms analyse transaction patterns to identify anomalies and flag potentially fraudulent activities in real-time. Additionally, banks employ deep learning for credit scoring, assessing the creditworthiness of individuals and businesses by analysing vast amounts of historical data. Customer service is also enhanced through the use of chatbots powered by deep learning, which provide personalised assistance and resolve queries swiftly. Furthermore, deep learning aids in risk management by predicting market trends and potential financial risks, enabling banks to make informed decisions and optimise their investment strategies. Overall, the integration of deep learning into banking processes not only streamlines operations but also enhances security and customer satisfaction.
What is an example of deep learning in banking?
An example of deep learning in banking is the use of neural networks to enhance customer service through chatbots. These AI-powered chatbots are trained using deep learning algorithms to understand and respond to customer queries in a more personalised and efficient manner. By analysing vast amounts of data, such as transaction histories and customer preferences, these chatbots can provide tailored recommendations, assist with account inquiries, and even detect potential fraud. This application of deep learning not only improves customer satisfaction but also helps banks streamline their operations and provide round-the-clock support to their clients.
Is deep learning good for finance?
The question of whether deep learning is beneficial for finance is a common one in the industry. Deep learning has proven to be a valuable tool for financial institutions, offering advanced analytical capabilities that can enhance decision-making processes. By leveraging deep learning algorithms, businesses can analyse vast amounts of data to predict market trends, manage risks, and detect fraudulent activities with high accuracy. The ability of deep learning to uncover complex patterns in financial data makes it a powerful asset for improving efficiency and driving strategic outcomes in the finance sector.
What machine learning is used in finance?
In the realm of finance, various machine learning techniques are utilised to extract valuable insights and drive informed decision-making processes. Specifically, in the context of deep learning, neural networks play a crucial role in analysing complex financial data sets. These neural networks are adept at recognising patterns, trends, and anomalies within financial data, enabling institutions to make accurate predictions on market movements, risk assessment, fraud detection, and portfolio optimisation. By leveraging machine learning algorithms such as neural networks, the finance industry can enhance its analytical capabilities and stay ahead in a rapidly evolving market landscape.
How is deep learning used in finance?
Deep learning is utilised in finance for a variety of critical applications. One prominent use case involves predictive analytics, where deep learning algorithms analyse historical data to forecast market trends, stock prices, and risk factors with a high degree of accuracy. This enables financial institutions to make informed investment decisions, manage risks effectively, and optimise their portfolios. Additionally, deep learning plays a crucial role in fraud detection by identifying anomalies in financial transactions and detecting potential fraudulent activities in real-time. By leveraging the power of deep learning technology, the finance industry can enhance decision-making processes, improve operational efficiency, and mitigate risks more effectively.