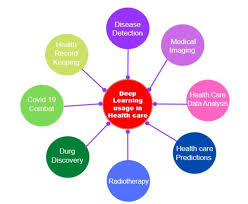
Advancements in Deep Learning Applications for Healthcare
Deep Learning in Healthcare
Deep learning, a subset of artificial intelligence (AI), has been revolutionizing various industries, including healthcare. Its ability to process vast amounts of data and identify complex patterns has made it a valuable tool in improving patient care, diagnosis, treatment, and medical research.
In healthcare, deep learning algorithms are being used to analyse medical images such as X-rays, MRIs, and CT scans with incredible accuracy. By training these algorithms on large datasets of images, they can detect abnormalities and assist healthcare professionals in diagnosing conditions like cancer, fractures, and other diseases at an early stage.
Moreover, deep learning models can predict patient outcomes based on their medical history, genetic information, and other factors. This predictive analytics helps doctors make informed decisions about treatment plans and interventions.
Another significant application of deep learning in healthcare is drug discovery. By analysing molecular structures and biological data, deep learning algorithms can accelerate the process of identifying potential drug candidates for various diseases.
Despite its promising potential, the implementation of deep learning in healthcare comes with challenges such as data privacy concerns, regulatory compliance, and ethical considerations. It is crucial for healthcare providers to ensure that patient data is handled securely and that the algorithms are transparent and unbiased.
As technology continues to advance, deep learning will play an increasingly important role in transforming the healthcare industry. By harnessing the power of AI-driven solutions, we can improve patient outcomes, enhance medical research capabilities, and ultimately revolutionize the way we deliver healthcare services.
Seven Benefits of Deep Learning in Healthcare: From Diagnostic Accuracy to Optimised Operations
- Improved diagnostic accuracy
- Early detection of diseases
- Personalised treatment plans
- Efficient medical image analysis
- Enhanced patient outcomes
- Accelerated drug discovery process
- Optimised healthcare operations
Challenges of Deep Learning in Healthcare: Privacy, Bias, Costs, and Compliance Issues
- Data privacy concerns related to storing and sharing sensitive patient information.
- Risk of bias in algorithms leading to inaccurate diagnoses or treatment recommendations.
- Complexity of deep learning models may make them difficult to interpret and explain to healthcare professionals.
- High costs associated with implementing and maintaining deep learning systems in healthcare facilities.
- Potential for overreliance on technology, reducing the human touch and personalised care in patient interactions.
- Regulatory challenges in ensuring compliance with data protection laws and healthcare regulations.
Improved diagnostic accuracy
One of the key advantages of incorporating deep learning into healthcare is the significant enhancement in diagnostic accuracy. Deep learning algorithms can analyse medical images and patient data with unparalleled precision, leading to more reliable and timely diagnoses. By leveraging vast datasets and complex pattern recognition capabilities, deep learning technologies empower healthcare professionals to detect subtle abnormalities and identify diseases at earlier stages, ultimately improving patient outcomes and prognosis. This boost in diagnostic accuracy not only benefits individual patients by enabling prompt and accurate treatment but also contributes to advancing medical research and enhancing overall healthcare quality.
Early detection of diseases
One of the key advantages of implementing deep learning in healthcare is its capability for early detection of diseases. By analysing vast amounts of medical data, including images and patient records, deep learning algorithms can identify subtle patterns and anomalies that may indicate the presence of a disease at its initial stages. This early detection enables healthcare professionals to intervene promptly, initiate treatment plans sooner, and potentially improve patient outcomes by addressing the disease in its early and more manageable phases.
Personalised treatment plans
One of the significant benefits of implementing deep learning in healthcare is the ability to create personalised treatment plans for patients. By analysing vast amounts of patient data, including medical history, genetic information, and treatment outcomes, deep learning algorithms can generate tailored treatment strategies that are specifically designed to meet the individual needs of each patient. This personalised approach not only improves the effectiveness of treatments but also minimises the risk of adverse reactions, leading to better patient outcomes and overall healthcare quality.
Efficient medical image analysis
One of the significant advantages of deep learning in healthcare is its ability to conduct efficient medical image analysis. Deep learning algorithms can process vast amounts of medical images such as X-rays, MRIs, and CT scans with remarkable accuracy and speed. By training these algorithms on large datasets, healthcare professionals can quickly and accurately identify abnormalities, diagnose conditions, and provide timely treatment to patients. This streamlined approach to medical image analysis not only improves diagnostic accuracy but also enhances the overall efficiency of healthcare delivery, ultimately leading to better patient outcomes.
Enhanced patient outcomes
Deep learning in healthcare has the remarkable ability to enhance patient outcomes by leveraging advanced algorithms to analyse vast amounts of data and provide more accurate diagnoses and treatment plans. By harnessing the power of deep learning, healthcare professionals can make informed decisions based on predictive analytics, leading to earlier detection of diseases, personalised treatment approaches, and ultimately improved patient care. This transformative technology not only increases the efficiency of healthcare delivery but also contributes to better health outcomes for patients, highlighting the significant impact that deep learning can have on enhancing overall patient well-being.
Accelerated drug discovery process
One of the significant advantages of incorporating deep learning in healthcare is its ability to accelerate the drug discovery process. By leveraging deep learning algorithms to analyse vast amounts of molecular data and biological information, researchers can identify potential drug candidates for various diseases more efficiently than traditional methods. This accelerated drug discovery process not only speeds up the development of new treatments but also holds the promise of addressing unmet medical needs and improving patient outcomes in a timely manner.
Optimised healthcare operations
Deep learning in healthcare offers the significant benefit of optimising healthcare operations. By analysing vast amounts of data, deep learning algorithms can streamline administrative processes, improve resource allocation, and enhance operational efficiency within healthcare facilities. This optimisation allows healthcare providers to deliver better quality care to patients, reduce waiting times, and make more informed decisions that ultimately lead to improved patient outcomes. The ability of deep learning to identify patterns and trends in data enables healthcare organisations to operate more effectively and provide timely and personalised care to those in need.
Data privacy concerns related to storing and sharing sensitive patient information.
Data privacy concerns are a significant challenge when it comes to implementing deep learning in healthcare. The storage and sharing of sensitive patient information, such as medical histories and genetic data, require stringent security measures to prevent unauthorized access and breaches. Ensuring that patient data is handled with the utmost confidentiality is paramount, as any compromise could lead to serious ethical and legal ramifications. Additionally, there is a need for robust regulatory frameworks to govern how this data is collected, stored, and used, ensuring that patients’ rights are protected while still enabling the advancements that deep learning can bring to medical research and treatment.
Risk of bias in algorithms leading to inaccurate diagnoses or treatment recommendations.
One significant concern regarding the use of deep learning in healthcare is the risk of bias in algorithms, which can result in inaccurate diagnoses or treatment recommendations. If the training data used to develop these algorithms is not diverse or representative enough, it may lead to skewed results that disproportionately impact certain patient groups. This bias could potentially exacerbate existing healthcare disparities and compromise the quality of care delivered to patients. Healthcare providers must remain vigilant in addressing and mitigating algorithmic bias to ensure that deep learning technologies contribute positively to patient outcomes and overall healthcare quality.
Complexity of deep learning models may make them difficult to interpret and explain to healthcare professionals.
The complexity of deep learning models in healthcare presents a significant challenge as it may render them difficult to interpret and explain to healthcare professionals. Due to the intricate nature of these algorithms and the layers of abstraction involved, understanding how they arrive at specific conclusions or predictions can be a daunting task for medical practitioners. This lack of interpretability raises concerns about trust, accountability, and the potential for errors in decision-making processes within the healthcare setting. Efforts to enhance transparency and develop methods for explaining deep learning outputs are essential to ensure that these advanced technologies can be effectively integrated into clinical practice while maintaining patient safety and ethical standards.
High costs associated with implementing and maintaining deep learning systems in healthcare facilities.
The high costs associated with implementing and maintaining deep learning systems in healthcare facilities pose a significant challenge for widespread adoption. From acquiring the necessary hardware and software to training staff and ensuring compliance with regulatory standards, the financial burden of integrating deep learning technology can be prohibitive for many healthcare institutions. These costs not only include the initial investment but also ongoing expenses related to system upgrades, maintenance, and data security measures. As a result, the affordability and sustainability of deploying deep learning solutions in healthcare settings remain a pressing concern that must be addressed to fully realize the potential benefits of this innovative technology.
Potential for overreliance on technology, reducing the human touch and personalised care in patient interactions.
One significant con of deep learning in healthcare is the potential for overreliance on technology, which may lead to a reduction in the human touch and personalised care during patient interactions. As healthcare professionals increasingly rely on AI-driven solutions for diagnosis and treatment decisions, there is a risk of dehumanising patient care and diminishing the importance of empathy and individualised attention. The human element in healthcare, including communication, compassion, and understanding, plays a crucial role in building trust between patients and providers. Therefore, it is essential to strike a balance between leveraging the benefits of deep learning technology and maintaining the personalised care that patients deserve.
Regulatory challenges in ensuring compliance with data protection laws and healthcare regulations.
One significant con of deep learning in healthcare is the regulatory challenges associated with ensuring compliance with data protection laws and healthcare regulations. As deep learning algorithms rely on vast amounts of sensitive patient data to function effectively, healthcare providers must navigate stringent legal frameworks designed to protect patient privacy and ensure data security. This includes adhering to regulations such as the General Data Protection Regulation (GDPR) in the UK and Europe, which imposes strict guidelines on how personal data can be collected, stored, and processed. Additionally, healthcare providers must ensure that their AI systems meet industry-specific standards and obtain necessary approvals from regulatory bodies before deployment. These challenges can slow down the adoption of deep learning technologies in healthcare and require significant investment in legal expertise and compliance measures to mitigate potential risks.