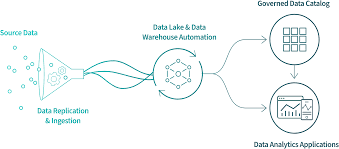
Unveiling the Power of Data: Exploring Data Science Insights
Data Science Insights: Unveiling the Power of Data
In today’s digital age, data has become the lifeblood of businesses and organizations across various industries. From e-commerce giants to healthcare providers, decision-makers are increasingly relying on data-driven insights to gain a competitive edge and make informed choices. This is where the field of data science comes into play, unlocking the untapped potential hidden within vast amounts of information.
Data science is a multidisciplinary field that combines statistical analysis, machine learning algorithms, and domain expertise to extract valuable insights from complex datasets. By leveraging advanced analytics techniques, data scientists can uncover patterns, trends, and correlations that may not be apparent at first glance.
One of the primary goals of data science is to transform raw data into actionable knowledge. By harnessing the power of statistical models and algorithms, experts can identify key drivers behind business outcomes or predict future trends with remarkable accuracy. These insights can then be used to optimize processes, enhance decision-making capabilities, and drive innovation.
In the realm of business intelligence, data science has revolutionized how organizations operate. Traditional methods of decision-making based on intuition or limited information have given way to evidence-based strategies driven by data-driven insights. With access to comprehensive datasets and powerful analytical tools, businesses can now make more informed choices that align with their goals and objectives.
The impact of data science extends far beyond business applications. In healthcare, for instance, it has proven instrumental in disease prediction and prevention. By analyzing patient records and medical research databases, data scientists can identify risk factors for certain conditions and develop personalized treatment plans. This not only improves patient outcomes but also helps healthcare providers allocate resources more efficiently.
Moreover, data science plays a crucial role in tackling social challenges such as climate change or urban planning. By analyzing environmental sensor data or urban mobility patterns, experts can devise sustainable solutions that minimize environmental impact while optimizing resource allocation.
Despite its immense potential, harnessing the power of data science requires a combination of technical expertise, domain knowledge, and ethical considerations. Data scientists must navigate complex ethical questions related to privacy, bias, and fairness when collecting and analyzing data. Transparency and accountability are paramount to ensure that the insights derived from data science are used responsibly and for the benefit of society as a whole.
In conclusion, data science is transforming how we understand and utilize information. By unlocking the power of data, businesses can gain a competitive advantage, healthcare providers can improve patient outcomes, and society as a whole can address pressing challenges. As the field continues to evolve, it is crucial for organizations to embrace data-driven decision-making and invest in the talent and resources needed to unlock the full potential of data science insights.
9 Essential Tips for Gaining Data Science Insights
- Invest in the right data science tools and technologies to help you gain insights quickly.
- Use data visualisation techniques to help make your insights easier to understand and interpret.
- Utilise machine learning algorithms such as decision trees, neural networks, and random forests to uncover new patterns in your data.
- Leverage natural language processing (NLP) technology to extract valuable information from unstructured text sources such as customer reviews or emails.
- Make sure you have a good understanding of statistics so that you can accurately interpret the results of your data analysis projects.
- Develop an iterative approach when analysing large datasets – start with a basic model and then refine it as needed based on insights gained from further analysis or feedback from stakeholders or customers.
- Stay up-to-date with the latest trends in data science, including new methods for collecting, storing, managing, and analysing data efficiently and effectively .
- Understand how different types of data can be used together to gain deeper insights into business processes or customer behaviour .
- Look for ways to automate parts of the process where possible , so that you can focus more time on gaining meaningful insights from your data
Invest in the right data science tools and technologies to help you gain insights quickly.
Invest in the Right Data Science Tools: Unlocking Insights at Lightning Speed
In the fast-paced world of data science, time is of the essence. As businesses strive to gain a competitive edge and make informed decisions, investing in the right tools and technologies becomes paramount. With the right resources at your disposal, you can unlock valuable insights quickly, giving you a significant advantage in today’s data-driven landscape.
Data science tools and technologies have evolved significantly in recent years, offering powerful capabilities that simplify complex tasks and accelerate the analysis process. From advanced analytics platforms to machine learning frameworks, these tools empower data scientists to extract meaningful insights from vast datasets with ease.
By investing in cutting-edge data science tools, you can streamline your workflow and enhance productivity. These tools often come equipped with intuitive interfaces and pre-built algorithms that reduce the time spent on repetitive tasks. This allows data scientists to focus on higher-level analysis and interpretation, ultimately leading to quicker insights.
Furthermore, modern data science tools enable seamless integration with various data sources. Whether you’re working with structured databases or unstructured text documents, these technologies provide efficient ways to ingest and process diverse datasets. This versatility enables comprehensive analysis across multiple dimensions, giving you a holistic view of your data.
Another key benefit of investing in the right tools is their ability to handle large-scale computations efficiently. Big data has become ubiquitous in today’s digital landscape, posing challenges for traditional computing infrastructures. However, advanced data science tools leverage distributed computing frameworks that can handle massive volumes of data effortlessly. This scalability ensures that you can extract insights from even the most extensive datasets without compromising performance.
Moreover, investing in robust data science technologies provides access to state-of-the-art machine learning algorithms and models. These algorithms automate complex analytical processes, allowing for faster model training and deployment. By leveraging these pre-built models or building upon them with your own customizations, you can expedite insight generation and make real-time predictions.
However, it’s essential to invest wisely and choose tools that align with your specific needs and goals. Consider factors such as scalability, ease of use, compatibility with existing systems, and the level of support provided by the tool’s developers. Conduct thorough research and seek recommendations from experts in the field to ensure you make informed decisions.
In conclusion, investing in the right data science tools and technologies is crucial for gaining insights quickly in today’s data-driven world. These tools streamline workflows, handle large-scale computations efficiently, and provide access to advanced analytics capabilities. By making strategic investments in this area, businesses can unlock valuable insights at lightning speed, giving them a competitive advantage and empowering data-driven decision-making.
Use data visualisation techniques to help make your insights easier to understand and interpret.
Use Data Visualization Techniques to Enhance Data Science Insights
In the world of data science, the ability to extract meaningful insights from complex datasets is crucial. However, presenting these insights in a way that is easily understandable and interpretable by stakeholders can be equally important. This is where the power of data visualization techniques comes into play.
Data visualization involves representing data in visual formats such as charts, graphs, or maps. By transforming raw numbers into visual representations, data scientists can effectively communicate patterns, trends, and relationships to non-technical audiences. This enables stakeholders to grasp complex concepts quickly and make informed decisions based on the insights presented.
One of the key advantages of data visualization is its ability to simplify complex information. Instead of overwhelming stakeholders with rows and columns of numbers or lengthy reports, visualizations provide a concise and intuitive representation of the data. Visual elements such as colours, shapes, and sizes can be used strategically to highlight important patterns or outliers within the dataset.
Moreover, data visualization allows for exploration and interactive analysis. With interactive visualizations, users can drill down into specific areas of interest or filter data based on different variables. This empowers stakeholders to explore the underlying patterns themselves and gain a deeper understanding of the insights presented.
Another benefit of using data visualization techniques is their ability to reveal hidden patterns or correlations that may not be apparent through traditional numerical analysis alone. By representing data visually, relationships between variables can become more evident, enabling data scientists to uncover valuable insights that might have otherwise gone unnoticed.
Furthermore, visualizations facilitate effective storytelling. By presenting insights in a visually compelling manner, data scientists can engage their audience and convey a narrative around the findings. This helps stakeholders connect with the information on an emotional level and increases their willingness to act upon the insights presented.
When it comes to choosing appropriate visualization techniques for your data science project, it’s essential to consider factors such as the type of data being analyzed and the intended audience. Different types of visualizations, such as bar charts, scatter plots, or heatmaps, can be used to represent different types of data and convey specific messages effectively.
In conclusion, incorporating data visualization techniques into your data science workflow can greatly enhance the understanding and interpretation of insights. By transforming complex information into visually appealing representations, stakeholders can grasp the key findings quickly and make informed decisions. As data continues to grow in volume and complexity, leveraging the power of data visualization will become increasingly vital for effective communication and decision-making in the field of data science.
Utilise machine learning algorithms such as decision trees, neural networks, and random forests to uncover new patterns in your data.
Unleashing the Power of Machine Learning Algorithms in Data Science Insights
In the rapidly evolving field of data science, machine learning algorithms have emerged as powerful tools to extract valuable insights from complex datasets. Among these algorithms, decision trees, neural networks, and random forests stand out as key players in uncovering new patterns and relationships within data.
Decision trees are intuitive models that mimic human decision-making processes. By splitting data into branches based on specific features, decision trees can reveal important variables and their impact on outcomes. These algorithms excel at identifying key factors that influence a particular outcome or behavior. With their visual representation and interpretability, decision trees offer valuable insights into complex decision-making scenarios.
Neural networks, on the other hand, are inspired by the structure of the human brain. Comprised of interconnected nodes (neurons), these algorithms can learn from vast amounts of data to recognize patterns and make predictions. Neural networks excel at handling unstructured data such as images or text, where traditional statistical models may fall short. Their ability to identify intricate relationships within data makes them a valuable tool for uncovering hidden insights.
Random forests combine the power of multiple decision trees to deliver robust results. By creating an ensemble of individual tree models, random forests reduce overfitting and improve generalization capabilities. This algorithm is particularly effective when dealing with large datasets with numerous variables. Random forests can handle missing values and noisy data while maintaining high accuracy levels in predicting outcomes.
By utilizing these machine learning algorithms in your data analysis process, you can unlock new patterns and relationships that may have remained hidden otherwise. These tools offer a deeper understanding of your data by enabling you to identify crucial variables impacting your business outcomes or research objectives.
However, it’s important to note that employing machine learning algorithms requires careful consideration and expertise. The selection and fine-tuning of these algorithms depend on the nature of your data and research questions. It is essential to understand their limitations and potential biases to ensure reliable and meaningful insights.
In conclusion, machine learning algorithms such as decision trees, neural networks, and random forests have revolutionized the field of data science. By leveraging their power, you can uncover valuable patterns and relationships within your data, leading to informed decision-making and innovation. Embracing these algorithms as part of your data analysis toolkit will undoubtedly enhance your ability to extract meaningful insights from complex datasets.
Leverage natural language processing (NLP) technology to extract valuable information from unstructured text sources such as customer reviews or emails.
Unlocking the Power of Natural Language Processing (NLP) in Data Science
In the realm of data science, one of the most powerful tools at our disposal is natural language processing (NLP) technology. With its ability to extract valuable information from unstructured text sources, such as customer reviews or emails, NLP has revolutionized how we analyze and understand textual data.
Traditionally, analyzing unstructured text sources was a daunting task. The sheer volume of information made it difficult to manually extract meaningful insights. However, with NLP, data scientists can now automate this process and uncover valuable nuggets of information that were once hidden within vast amounts of text.
By leveraging NLP techniques, businesses can gain a deeper understanding of their customers’ sentiments and preferences. For example, by analyzing customer reviews using sentiment analysis algorithms, companies can identify patterns in feedback and gain insights into what drives customer satisfaction or dissatisfaction. This information can then be used to improve products or services and enhance the overall customer experience.
Moreover, NLP enables organizations to extract key entities or topics from large volumes of text data. This allows them to identify emerging trends or topics of interest within customer feedback or industry news. By understanding these trends in real-time, businesses can stay ahead of the curve and make informed decisions based on up-to-date information.
Another application of NLP is in email analysis. By automatically categorizing and extracting relevant information from emails, organizations can streamline their workflows and improve efficiency. For instance, using email classification algorithms powered by NLP, companies can automatically route customer inquiries to the appropriate department or flag urgent issues for immediate attention. This not only saves time but also ensures that important messages are not overlooked.
However, it is important to note that leveraging NLP technology comes with its own set of challenges. Textual data often contains nuances such as sarcasm or ambiguity that can be difficult for machines to interpret accurately. Additionally, privacy concerns must be addressed when dealing with sensitive information contained within emails or customer reviews.
To overcome these challenges, data scientists continuously refine NLP models and algorithms, ensuring they are trained on diverse datasets to improve accuracy and mitigate bias. Furthermore, organizations must implement robust data governance practices to protect customer privacy and ensure compliance with relevant regulations.
In conclusion, leveraging NLP technology in data science allows us to unlock valuable insights from unstructured text sources. By automating the analysis of customer reviews or emails, businesses can gain a deeper understanding of their customers’ sentiments and preferences. This knowledge can drive improvements in products, services, and overall customer satisfaction. However, it is essential to address the challenges associated with NLP, such as interpreting nuanced language and safeguarding privacy. With careful consideration and implementation, NLP can truly revolutionize how we extract valuable information from unstructured text sources in the world of data science.
Make sure you have a good understanding of statistics so that you can accurately interpret the results of your data analysis projects.
The Importance of Statistical Understanding in Data Science Insights
In the world of data science, a solid understanding of statistics is essential for accurately interpreting the results of data analysis projects. Statistics provides the foundation for making sense of complex datasets and extracting meaningful insights. Let’s explore why having a good grasp of statistics is crucial for data scientists.
Firstly, statistics enables us to understand the inherent variability within data. Every dataset contains some level of randomness and uncertainty, and statistical techniques help us quantify and analyze this variability. By applying statistical concepts such as probability distributions, hypothesis testing, and confidence intervals, data scientists can assess the reliability and significance of their findings.
Moreover, statistical knowledge allows us to make informed decisions based on evidence rather than intuition. When conducting data analysis projects, it’s important to distinguish between correlation and causation. Statistics helps us identify meaningful relationships between variables while avoiding false conclusions or spurious connections. Understanding statistical concepts like regression analysis or experimental design empowers data scientists to draw reliable insights from their analyses.
Furthermore, statistics aids in evaluating the performance and accuracy of predictive models. Machine learning algorithms are commonly used in data science to make predictions or classifications based on historical patterns in the data. However, without a solid understanding of statistical measures such as accuracy, precision, recall, or F1 score, it becomes challenging to assess how well these models perform on unseen data. Statistical evaluation metrics provide valuable insights into model performance and guide decision-making processes.
Additionally, statistical expertise allows for effective communication with stakeholders who may not have a strong background in data science. As a data scientist, being able to explain your findings in a clear and understandable manner is crucial when presenting insights to non-technical audiences. A solid understanding of statistics helps you convey complex ideas using appropriate visualizations or simplified explanations that resonate with your audience.
In conclusion, having a good understanding of statistics is fundamental for accurately interpreting the results of data analysis projects in the field of data science. It enables data scientists to assess the reliability and significance of their findings, make evidence-based decisions, evaluate predictive models, and effectively communicate insights to stakeholders. By honing statistical knowledge alongside technical skills, data scientists can unlock the full potential of data science insights and drive impactful outcomes in various industries.
Develop an iterative approach when analysing large datasets – start with a basic model and then refine it as needed based on insights gained from further analysis or feedback from stakeholders or customers.
Developing an Iterative Approach: Unleashing the Power of Data Science Insights
In the realm of data science, analyzing large datasets can be a daunting task. The sheer volume and complexity of information can overwhelm even the most experienced data scientists. However, there is a valuable tip that can make this process more manageable and effective: developing an iterative approach.
The concept behind an iterative approach is simple yet powerful. Rather than attempting to build a perfect model from the start, data scientists begin with a basic model and continuously refine it based on insights gained from further analysis or feedback from stakeholders or customers.
Starting with a basic model allows data scientists to quickly gain an initial understanding of the dataset and identify any immediate patterns or trends. This initial analysis serves as a foundation upon which subsequent iterations can be built.
As further analysis is conducted, additional insights emerge, providing a clearer picture of the underlying patterns within the data. These insights may reveal new variables that should be considered or suggest adjustments to existing models. By incorporating these findings into subsequent iterations, data scientists can refine their models and improve their predictive capabilities.
Feedback from stakeholders or customers also plays a crucial role in this iterative process. Their perspectives and domain expertise can provide valuable insights that may not have been initially considered. Incorporating their feedback into subsequent iterations ensures that the final model aligns more closely with real-world needs and requirements.
The beauty of this iterative approach lies in its flexibility and adaptability. It allows for continuous improvement as new information becomes available or as project requirements evolve over time. By embracing this methodology, data scientists can avoid getting stuck in analysis paralysis and instead make meaningful progress towards extracting actionable insights from large datasets.
Furthermore, adopting an iterative approach promotes collaboration and knowledge sharing within teams. As each iteration builds upon previous ones, team members can contribute their expertise and collectively work towards refining the model. This collaborative environment fosters innovation and encourages diverse perspectives to shape the final outcome.
In conclusion, developing an iterative approach when analyzing large datasets is a valuable tip in the field of data science. By starting with a basic model and continuously refining it based on insights gained from further analysis or feedback from stakeholders or customers, data scientists can unlock the full potential of their data. This approach promotes flexibility, adaptability, and collaboration, ultimately leading to more accurate and actionable insights. So, embrace the power of iteration and unleash the true potential of your data science endeavors.
Stay up-to-date with the latest trends in data science, including new methods for collecting, storing, managing, and analysing data efficiently and effectively .
Staying Ahead: The Key to Harnessing the Power of Data Science Insights
In the ever-evolving world of data science, keeping up with the latest trends is essential to stay ahead of the curve. As new methods for collecting, storing, managing, and analyzing data emerge, it becomes crucial for data scientists and professionals to stay up-to-date with these advancements. By doing so, they can unlock the full potential of data science insights and drive innovation in their respective fields.
The field of data science is dynamic and constantly evolving. New techniques and technologies are being developed at a rapid pace, offering exciting opportunities for organizations to gain valuable insights from their data. By staying informed about the latest trends in data science, professionals can adopt innovative approaches that enhance their ability to collect, store, manage, and analyze data efficiently and effectively.
One key aspect to focus on is staying updated with new methods for collecting data. With advancements in technology, there are now numerous ways to gather information, such as through sensors, social media platforms, or IoT devices. By exploring these new avenues for data collection, organizations can access a wealth of valuable information that was previously untapped.
Additionally, staying informed about advancements in data storage is crucial. As datasets continue to grow exponentially in size and complexity, traditional storage methods may no longer suffice. Keeping up with new developments in cloud computing or distributed storage systems can help organizations efficiently store and access large volumes of data.
Managing vast amounts of data is another challenge that requires attention. Data governance frameworks and strategies are continually evolving to ensure compliance with regulations while maximizing the value derived from the collected information. Being aware of emerging practices in data management allows organizations to implement robust systems that maintain the integrity and security of their datasets.
Lastly, keeping abreast of cutting-edge analytical techniques is vital for extracting meaningful insights from collected data. From machine learning algorithms to artificial intelligence models, there are constant advancements that can enhance analysis capabilities. By staying up-to-date with these techniques, data scientists can uncover hidden patterns, predict future trends, and make accurate forecasts that drive informed decision-making.
In conclusion, staying up-to-date with the latest trends in data science is crucial for harnessing the power of data science insights. By being aware of new methods for collecting, storing, managing, and analyzing data efficiently and effectively, professionals can unlock the full potential of their datasets. Embracing innovation in data science allows organizations to gain a competitive edge, make informed decisions, and drive positive change in their industries. So, stay curious and keep exploring the ever-evolving world of data science!
Understand how different types of data can be used together to gain deeper insights into business processes or customer behaviour .
Unlocking Deeper Insights: Harnessing the Power of Different Data Types in Data Science
In the realm of data science, understanding how different types of data can be used together is a game-changer. By combining various sources and types of data, businesses can gain deeper insights into their processes and customer behavior, leading to more informed decision-making and enhanced outcomes.
Traditionally, businesses have relied on structured data, such as sales figures or customer demographics, to drive their strategies. While this type of data provides valuable insights, it only scratches the surface of what is possible. By incorporating unstructured data, such as social media posts or customer reviews, into the analysis, a more comprehensive picture emerges.
Unstructured data holds immense potential for uncovering hidden patterns and sentiments that may not be evident in structured datasets alone. By leveraging natural language processing and text mining techniques, businesses can extract valuable information from customer feedback or online conversations. This allows them to understand customer preferences, sentiment towards products or services, and even predict future trends.
Furthermore, integrating external datasets from various sources can enrich the analysis even further. For example, combining weather data with sales figures can reveal how weather conditions impact consumer behavior and inform marketing strategies accordingly. Similarly, merging demographic information with transactional data can help identify target markets and tailor campaigns more effectively.
By understanding how different types of data can complement each other, businesses gain a holistic view of their operations and customers. They can identify correlations between seemingly unrelated factors and make connections that were previously overlooked. This opens up opportunities for optimization across various business functions – from supply chain management to marketing campaigns.
However, it’s important to note that working with diverse datasets comes with its challenges. Data integration requires careful consideration of data quality assurance and standardization processes to ensure accuracy and consistency across sources. Additionally, privacy concerns must be addressed when handling sensitive information from multiple channels.
To harness the power of different data types effectively, organizations need to invest in robust data infrastructure and employ skilled data scientists who can navigate complex datasets. These experts must possess a deep understanding of statistical techniques, machine learning algorithms, and domain knowledge to extract meaningful insights.
In conclusion, combining different types of data is a crucial aspect of data science that can unlock deeper insights into business processes and customer behavior. By incorporating structured and unstructured data from multiple sources, businesses can gain a comprehensive understanding of their operations, identify trends, and make more informed decisions. However, it is essential to address challenges such as data quality assurance and privacy concerns to ensure the responsible use of diverse datasets. With the right expertise and infrastructure in place, organizations can leverage the power of different data types to drive innovation and achieve sustainable growth.
Look for ways to automate parts of the process where possible , so that you can focus more time on gaining meaningful insights from your data
Maximizing Data Science Insights: Automating for Efficiency
In the fast-paced world of data science, time is of the essence. As data scientists, we often find ourselves juggling multiple tasks, from data collection and cleaning to model development and analysis. With so much on our plates, it’s crucial to find ways to streamline our workflow and make the most of our valuable time. One effective strategy is to automate parts of the process where possible.
Automation in data science involves leveraging tools, scripts, and algorithms to perform repetitive or time-consuming tasks. By automating these aspects, we free up more time for what truly matters – gaining meaningful insights from our data.
One area where automation can be particularly beneficial is in data preprocessing. This stage often involves cleaning messy datasets, handling missing values, and transforming variables into suitable formats for analysis. By developing automated scripts or workflows that handle these tasks, we can save hours or even days of manual work. This not only increases efficiency but also reduces the risk of human error.
Another aspect that can be automated is model training and evaluation. Machine learning algorithms often require extensive experimentation with different hyperparameters and model architectures. Automating this process allows us to explore a wider range of possibilities in less time. We can use techniques like grid search or Bayesian optimization to systematically search through parameter spaces and identify optimal configurations.
Furthermore, automating the deployment and monitoring of models can enhance efficiency in real-world applications. By setting up pipelines or workflows that automatically update models with new data, we ensure that our insights remain up-to-date without continuous manual intervention. Additionally, monitoring tools can alert us if models start to underperform or encounter anomalies, allowing us to take timely corrective actions.
While automation offers numerous benefits in terms of efficiency and time savings, it’s important to strike a balance between automation and human expertise. Data science is not just about running algorithms; it requires critical thinking and domain knowledge to interpret results and draw meaningful insights. Therefore, it’s crucial to allocate sufficient time for analysis and interpretation, even when automation is in place.
In conclusion, automating parts of the data science process can significantly enhance efficiency and allow us to focus more on gaining meaningful insights from our data. By automating tasks like data preprocessing, model training, and deployment, we can save time, reduce errors, and explore a wider range of possibilities. However, it’s essential to maintain a balance between automation and human expertise to ensure accurate interpretation of results. Embracing automation in data science empowers us to make the most of our valuable time and deliver impactful insights that drive informed decision-making.