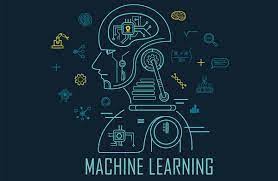
Exploring the Impact of Machine Learning in Hindi Language
The Impact of Machine Learning in Hindi Language
Machine learning, a subset of artificial intelligence, has been making significant advancements in various fields, including natural language processing. In recent years, the application of machine learning in the Hindi language has gained momentum, opening up new possibilities and opportunities for both researchers and industry professionals.
One of the key areas where machine learning is transforming the Hindi language is machine translation. With the help of sophisticated algorithms and neural networks, machine translation systems are becoming increasingly accurate in translating text from Hindi to other languages and vice versa. This has immense potential for breaking down language barriers and facilitating communication on a global scale.
Furthermore, machine learning is also being used to develop chatbots and virtual assistants that can interact with users in Hindi. These intelligent systems are capable of understanding natural language queries, providing relevant information, and even engaging in conversations with users. This not only enhances user experience but also opens up new avenues for customer service and support.
Moreover, machine learning algorithms are being employed to analyse large volumes of textual data in Hindi, enabling organisations to gain valuable insights and make informed decisions. From sentiment analysis to topic modelling, these algorithms can extract meaningful information from unstructured text data, helping businesses improve their operations and strategies.
In conclusion, the integration of machine learning in the Hindi language is revolutionising how we interact with technology and process linguistic data. As advancements continue to be made in this field, we can expect to see even more innovative applications that leverage the power of machine learning to enhance our understanding and use of the Hindi language.
Six Essential Tips for Enhancing Machine Learning Skills
- मशीन लर्निंग में डेटा की गुणवत्ता बहुत महत्वपूर्ण होती है। सुनिश्चित करें कि आपका डेटा साफ और सटीक हो।
- अलग-अलग एल्गोरिदम का प्रयोग करके देखें, क्योंकि हर समस्या के लिए एक ही समाधान काम नहीं करता।
- हाइपरपैरामीटर ट्यूनिंग से मॉडल की प्रदर्शन क्षमता बढ़ाई जा सकती है।
- ओवरफिटिंग से बचने के लिए क्रॉस-वैलिडेशन तकनीकों का उपयोग करें।
- फीचर इंजीनियरिंग पर ध्यान दें; अच्छे फीचर्स मॉडल की सटीकता को काफी हद तक प्रभावित कर सकते हैं।
- अपनी मशीन लर्निंग स्किल्स को बढ़ाने के लिए नियमित रूप से नई रिसर्च पढ़ें और प्रैक्टिकल प्रोजेक्ट्स पर काम करें।
मशीन लर्निंग में डेटा की गुणवत्ता बहुत महत्वपूर्ण होती है। सुनिश्चित करें कि आपका डेटा साफ और सटीक हो।
Quality of data plays a crucial role in machine learning. Ensuring that your data is clean and accurate is essential to the success of any machine learning project. By maintaining high-quality data, you can improve the performance and reliability of your machine learning models, ultimately leading to more accurate predictions and valuable insights. Therefore, it is imperative to pay close attention to the quality of data to maximise the effectiveness of machine learning algorithms.
अलग-अलग एल्गोरिदम का प्रयोग करके देखें, क्योंकि हर समस्या के लिए एक ही समाधान काम नहीं करता।
It is advisable to experiment with different algorithms in machine learning when working with Hindi language data, as not every problem has a one-size-fits-all solution. By trying out various algorithms, researchers and practitioners can identify the most suitable approach for a specific task or dataset, leading to more accurate results and better insights. This iterative process of testing different algorithms helps in understanding the nuances of the data and selecting the most effective model for addressing the unique challenges posed by each problem in Hindi language processing.
हाइपरपैरामीटर ट्यूनिंग से मॉडल की प्रदर्शन क्षमता बढ़ाई जा सकती है।
Hyperparameter tuning can significantly enhance the performance capability of a model. By adjusting the hyperparameters effectively, the model’s accuracy and efficiency can be improved, leading to better results and outcomes. This process plays a crucial role in fine-tuning machine learning models to achieve optimal performance levels in handling complex tasks and datasets.
ओवरफिटिंग से बचने के लिए क्रॉस-वैलिडेशन तकनीकों का उपयोग करें।
To avoid overfitting, utilise cross-validation techniques. By using cross-validation methods, you can assess the performance of your machine learning model more accurately and prevent it from memorising the training data too closely. This approach helps in evaluating the model’s generalisation ability and ensures that it can make reliable predictions on unseen data.
फीचर इंजीनियरिंग पर ध्यान दें; अच्छे फीचर्स मॉडल की सटीकता को काफी हद तक प्रभावित कर सकते हैं।
Focusing on feature engineering is crucial in machine learning; good features can significantly impact the accuracy of the model. The process of selecting and preparing relevant features plays a vital role in determining the performance and effectiveness of a machine learning model. By paying attention to feature engineering, we can enhance the predictive power of the model and improve its ability to extract meaningful patterns and insights from data.
अपनी मशीन लर्निंग स्किल्स को बढ़ाने के लिए नियमित रूप से नई रिसर्च पढ़ें और प्रैक्टिकल प्रोजेक्ट्स पर काम करें।
To enhance your machine learning skills, it is essential to regularly engage in reading new research and working on practical projects. By staying updated with the latest developments in the field and applying your knowledge to hands-on projects, you can deepen your understanding and proficiency in machine learning. Continuous learning and practical application are key to honing your skills and staying ahead in the ever-evolving landscape of machine learning.